이미지 약 20,000장을 사용하여 Tiny-YOLO를 학습시켰습니다. 하지만 기대했던 만큼 성능이 나오지 않았습니다. 딥러닝에서 데이터의 양이 많으면 많을수록 성능이 올라가는 것은 알려진 사실입니다. 원인을 파악하기 위해서 학습된 Tiny-YOLO 모델이 어떤 물체를 볼라드라고 탐지하는 분류했습니다. 실제 볼라드는 볼라드라고 잘 탐지했습니다. 하지만 카메라 화면에 보이는 큰 사각형, 자동차 범퍼, 문 등을 볼라드라고 탐지하는 것을 알게 되었습니다. (아쉽게도, 이 당시 촬영한 영상과 사진이 없습니다.) 그래서 Dataset을 들여다 보니, Dataset에 문제가 있는 것으로 추정했습니다. 15,000장은 인터넷에서 크롤링한 dataset이었는데, 사진 속 볼라드는 카메라로 확대해서 촬영됬거나 근접 촬영이 되었거나 화질이 좋지 못했습니다.
왼쪽의 인터넷 볼라드를 보면 굉장히 근접에서 볼라드만을 찍은 것을 알 수 있습니다. 하지만 카메라를 꺼내서 사람의 눈 높이에서 촬영하면 오른쪽 사진과 같이 나옵니다.
YOLO는 grid cell을 이용해서 cell마다 학습시키는 방법을 사용하므로 크기의 비율이 실제 사용하는 환경과 차이가 나면 안된다는 추정을 했습니다. 그리고 화질이 다르면 실제 이미지와 학습 이미지의 distributions이 달라지기 때문에 이 또한 성능이 낮게 나오는 이유라고 추정했습니다. 그래서 Dataset에서 화질이 1280*720(촬영 카메라 화질이 HD였기 때문에)이 아니고, 볼라드가 과하게 작고 큰 사진들을 모두 제거하니 약 5,000장의 이미지가 남았습니다. 그리고 다시 학습을 시키고 테스트를 해보니 이전보다 훤씬 성능이 좋았습니다. 같은 구간에서 핸드폰을 들고 테스트 했었습니다.
(테스트를 눈으로 확인만 하고 기록하지 않았습니다. 그래서 비슷한 상황을 보여주기 위해서 예시를 만들었습니다.)
이전 예시를 보면 바닥의 페인트, 차 앞 부분, 세워진 간판, 등 볼라드라고 탐지했습니다. 하지만 이후 예시는 화분을 볼라드라고 탐지했습니다. (직사각형에 흰색, 노란색, 검은색이면 볼라드라고 탐지하는 경우가 많습니다.)
4,000장으로 학습을 시킨 후, 실제 거리에서 테스트를 한 결과 100개의 볼라드 지점에서 100번 다 볼라드가 있다고 탐지를 했었습니다. 물론 이 볼라드는 원기둥형 볼라드입니다. 사격형, 반구형 볼라드는 34개 중에 21번 볼라드라고 탐지했습니다. 그 이유는 data부족이라고 생각합니다. 4,000의 90%는 저와 친구가 강남, 잠실, 서초 구역을 돌아다니면서 실제로 수집한 이미지 자료였습니다. 그런데 반구형, 사각형 볼라드는 원기둥형 보다 쉽게 찾을 수 없었습니다.
이렇게 영상의 정확도를 올리는 방법을 소개했습니다. 다음 포스트에서는 저희 어플의 다른 기능인 낙상 감지 기능을 적어보겠습니다.
English
I had trained Tiny-YOLO with about 20,000 images. I expected somehow high performance, but it was not. As you have known, more images, more performance. However, it did not work for me. To find what was a problem, i analyze which objects were classified as Bollard on camera preview. The Tiny-YOLO detected real bollard as bollard. But, it detected any big square and retangle, front part of car, door as bollard. (Sadly, i don't have any video and picture for you to show what was going wrong.) I looked dataset image long time, and i figured out there was a problem. I crawled 15,000 images from google, and i think they were bad for training because some images zoomed in bollard or had low solution.
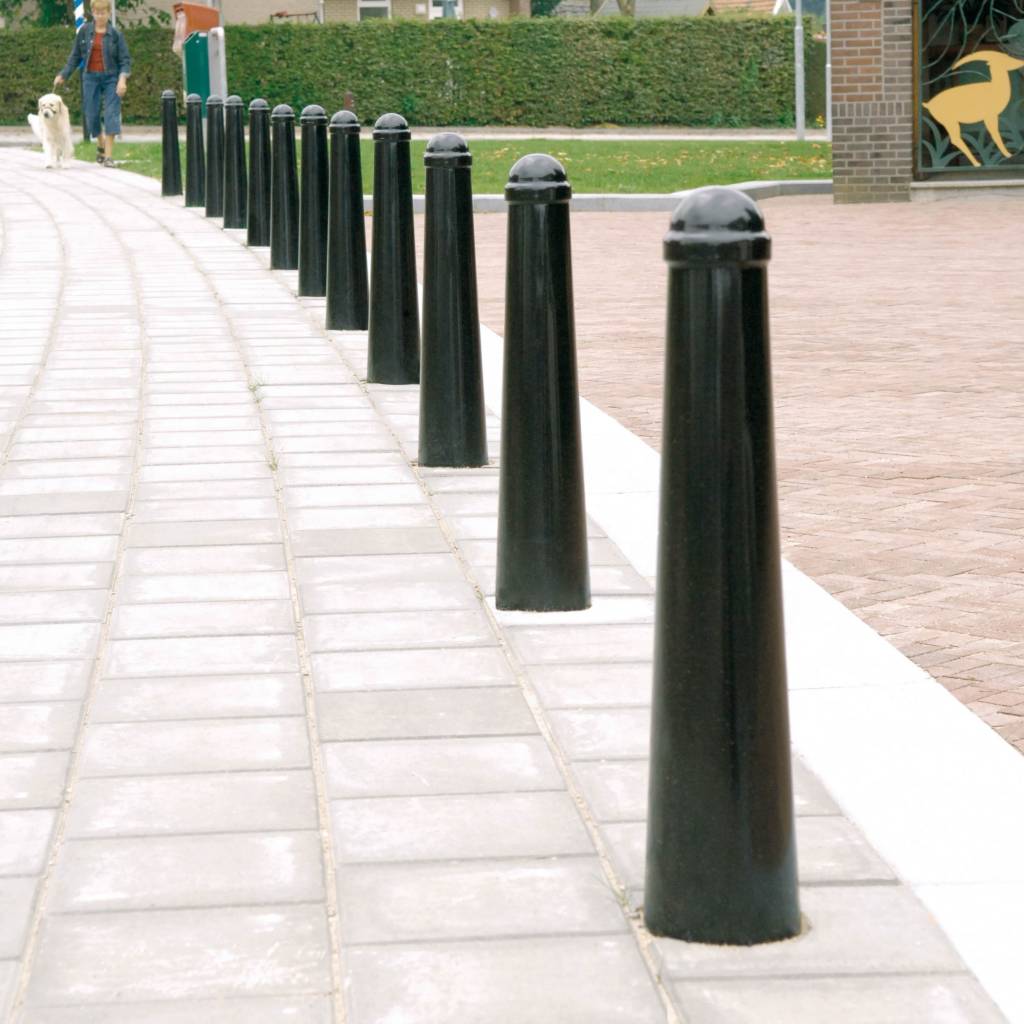
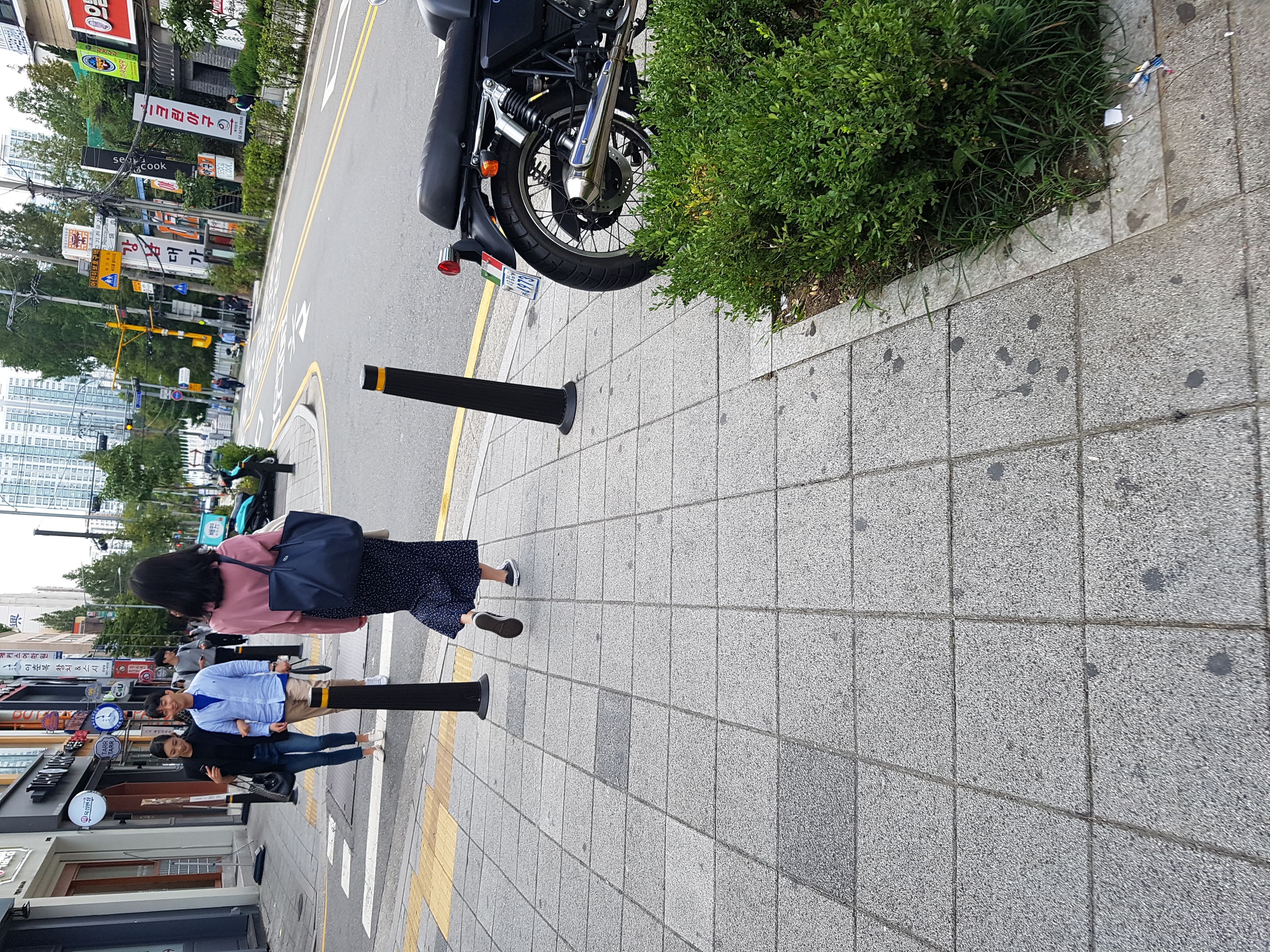
If you look at the Internet bollard on the left, you can see that only the bollards were taken in close proximity. In the other hand, if you took a picture at the same level of eyes, bollard will be like right image.
I presumed that the proportion of size between real bollard and train bollard should be same because YOLO is trained every cell. Moreover, if the solution would be different between real and train image, distributions are also different. So, I removed all image which were not 1280*720(because i used HD camera) and contained big and small bollard on. There were only 5,000 images left. I trained Tiny-YOLO again with them and it performed better than before. I tested it on the same street by phone camera.
(I only checked if it worked better or not. So, i made an similar example below to understand you. Hope it helps ;))
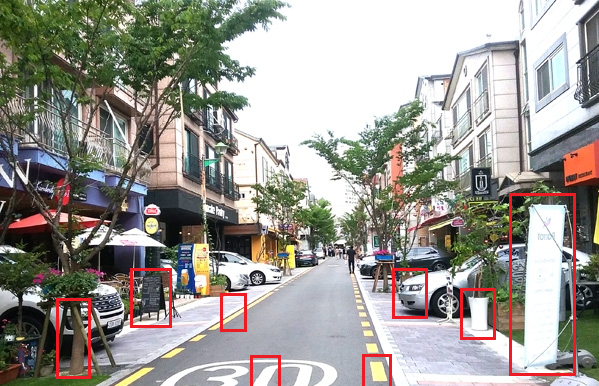
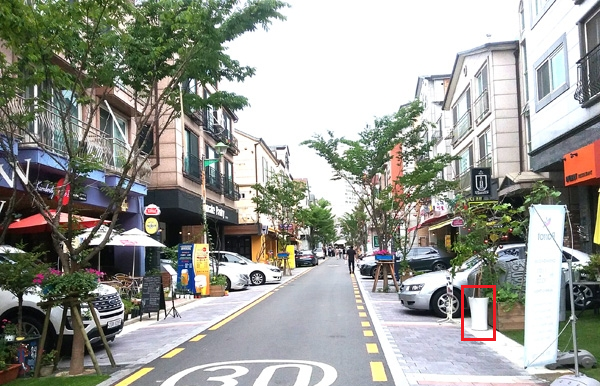
If you look left example, yellow painting, flowerpot, front of car, standing and tree trunk were detected as bollard. However, after trained again, flowerpot was detected as bollard. ( retangle shapes with white, yellow, black were often detected as Bollard )
After trained with 4,000 images, we had tested on streets and it detected 100 bollards at 100 bollards. But it was cylinder shape. On retangle and hemisphere shape, it detected 21 bollards at 34 bollards. I think the reason is the lack of data. We almost manually collected 4,000 images. When me and my friend had walked and taken pictures of bollard at Gangnam, Jamsil and seocho, we rarely saw square and hemisphere shpae bollard.
This post is for how to improve YOLO detection accuracy by my own experience. I will write about falling detector next post!
'Career > Barrier Free Application Development' 카테고리의 다른 글
총 정리 : 시작은 도전, 마무리는 성장 (3) | 2020.04.01 |
---|---|
기능: 근접 알림 센서 (4단계) (0) | 2020.04.01 |
기능: 낙상 감지(fall detector) - 낙상 알고리즘 (4단계) (0) | 2020.02.20 |
기능: 볼라드 탐지하기 (Bollard Detector) - Tiny YOLO 앱에 탑재하기(2단계) (12) | 2020.02.19 |
베리어 프리 앱 개발 콘테스트 (1단계) (0) | 2020.02.19 |